AIOps Now: Scaling Kubernetes With AI and Machine Learning
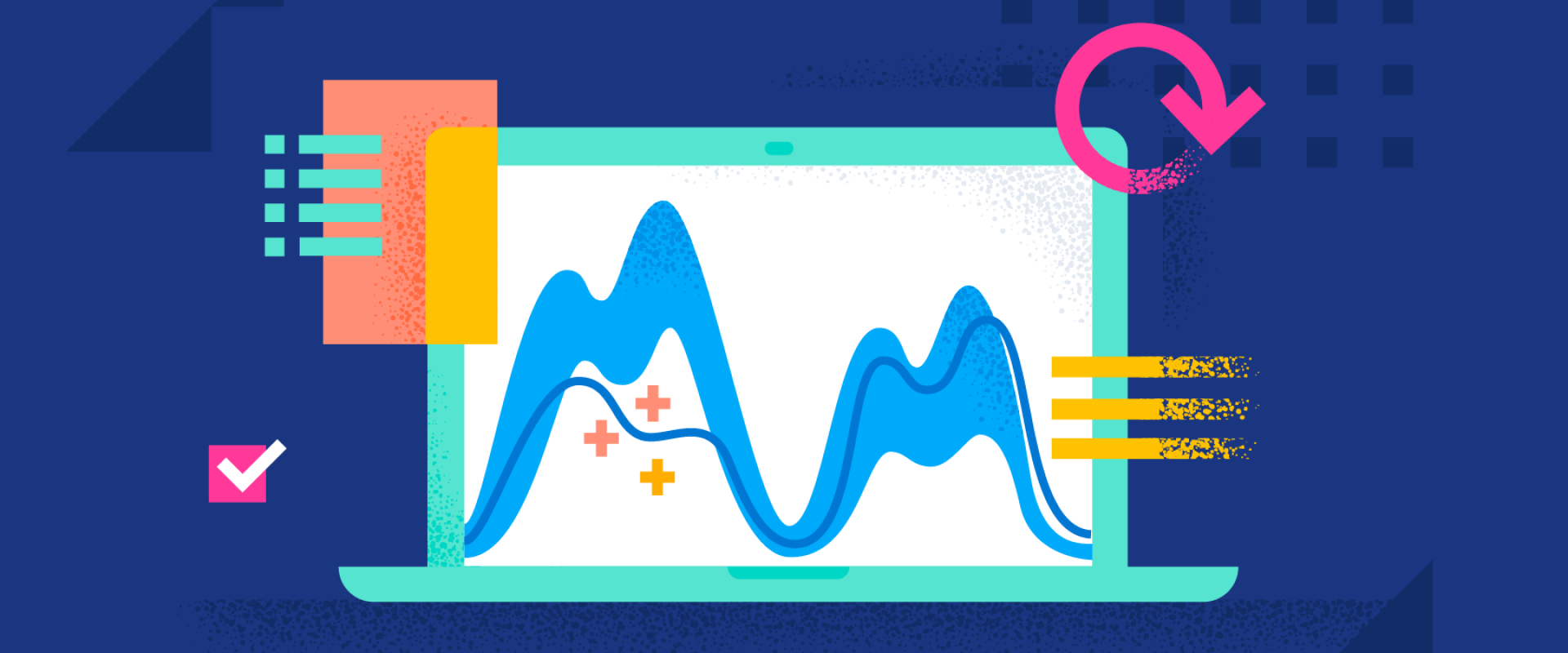
Using AI and digital twins, optimize Kubernetes apps and address SRE challenges with continuous learning for improved outcomes.
If you are a site reliability engineer (SRE) for a large Kubernetes-powered application, optimizing resources and performance is a daunting job. Some spikes, like a busy shopping day, are things you can broadly schedule, but, if done right, would require painstakingly understanding the behavior of hundreds of microservices and their interdependence that has to be re-evaluated with each new release — not a very scalable approach, let alone the monotony and resulting stress to the SRE. Moreover, there will always be unexpected peaks to respond to. Continually keeping tabs on performance and putting the optimal amount of resources in the right place is essentially impossible.
The way this is being solved now is through gross overprovisioning, or a combination of guesswork and endless alerts — requiring support teams to review and intervene. It’s simply not sustainable or practical, and certainly not scalable. But it’s just the kind of problem that machine learning and AI thrives on. We have spent the last decade dealing with such problems, and the arrival of the latest generation of AI tools such as generative AI has opened the possibility of applying machine learning to the real problems of the SRE to realize the promise of AIOps.
Turning Up the Compute Knob…to Be Safe
No matter how great your observability dashboard, the amount of data and the need for agility is just too much. You have to provision adequate resources to achieve the desired response times and error rates. It is not unusual for people in this role to peg compute utilization at 30 percent “to be safe” and be prepared to monitor hundreds of microservices to ensure the desired service-level agreement (SLA) is achieved. The end result is costly — not just from compute resources, but also DevOps resources dedicated to maintaining the SLA.
It seems that, for all it has brought us, Kubernetes has gone beyond the comprehension of those charged with operating it. Horizontal pod autoscaling (HPA) and reactive scaling solutions still leave the SREs guessing at what level to set the CPU utilization threshold that would work for various traffic loads and service graph dependencies. Traffic does not have a linear relationship to microservice loading and thus to performance, and that is not the only reason to change the states of the application deployment. SREs are also monitoring issues like temperature, faults, and latency.
For a typical Kubernetes application, there are on average several hundreds of microservices. Furthermore, each microservice is dependent on other microservices in a web of interconnected relationships with other microservices. It is not easy for a person to view and understand it all and then make detailed changes and do this repeatedly for every release of each microservice every week. SREs figuratively “turn up the compute knob” and hope that it improves whatever has dropped below the service-level objective (SLO). But, the reality is that it is useless to increase resources at a microservice which is dependent on another microservice, which is actually the bottleneck.
An Ideal Use Case for AI
In 2024, when someone says AI, the next thought is almost inevitably ChatGPT. ChatGPT is generative AI that selects the best next word. While the architecture required for a strong AIOps platform is very different from ChatGPT (more on that later), the goal is similar — choose the best next state for the application.
The intricately interconnected ecosystems of modern microservice applications are too big and complex for the SRE team to comprehend in detail and make those decisions. Most efforts to autoscale these applications fail to take into account the nuanced requirements and performance needs of individual services. I’ve been hearing about this problem continuously for over 20 years (starting with the L5 network load balancer we invented at Arrowpoint Communications).
The Digital Twin Goes Through the Paces
Training data is the fuel for AI. To teach an application to operate a mission critical Kubernetes instance, we need to develop good information about how the performance can be optimized. Digital twins have been used for decades in multiple fields including manufacturing and racing to help people recreate a digital equivalent of the real subject to study its behavior. In our case, we use performance metrics to build a digital twin of each microservice.
In reinforcement learning (RL), digital twins are used to create a simulation environment to generate an observation space in which a model can be trained to discover and learn the best paths (also known as "trajectories") to guide the system to states that have the desired target properties in terms of cost, performance, etc. In our case, we use proximal policy optimization (PPO) as the RL training algorithm. Our approach is service-graph aware to take into account the dependencies of microservices that impact scaling. Ultimately, we will have a model-free network that is continually learning based on operational experience.
Better Responsiveness and Ongoing Improvement
Kubernetes has come a long way. There is extensive tool-level automation, but not a lot of effective system-level automation. Perhaps that has a lot to do with the vast amount of activity within a Kubernetes instance. We boiled the problem down to deciding the best next state for the application.
People have been playing with generative AI that can produce words and images for a general audience. We are seeing how the same technology can transform our digital experience.
For SREs Now and Developers of the Future
SREs today could benefit from a transformation. Talking to SRE teams, we have learned that they are asked to contribute to their own SLOs and they simply don’t know where to begin. It seems that the complexity of Kubernetes has outpaced the ability of humans alone to operate it.
Looking ahead, applying AIOps models and moving toward autonomous infrastructure can allow for a new level of complexity and scale for microservices applications.
The Future Is Cloud-Native: Are You Ready?
Embrace the next generation of application development with cloud-native architecture.
Why Go Cloud-Native?
Cloud-native technologies empower us to produce increasingly larger and more complex systems at scale. It is a modern approach to designing, building, and deploying applications that can fully capitalize on the benefits of the cloud. The goal is to allow organizations to innovate swiftly and respond effectively to market demands.
Agility and Flexibility
Organizations often migrate to the cloud for the enhanced agility and the speed it offers. The ability to set up thousands of servers in minutes contrasts sharply with the weeks it typically takes for on-premises operations. Immutable infrastructure provides confidence in configurable and secure deployments and helps reduce time to market.
Scalable Components
Cloud-native applications are more than just hosting the applications on the cloud. The approach promotes the adoption of microservices, serverless, and containerized applications, and involves breaking down applications into several independent services. These services integrate seamlessly through APIs and event-based messaging, each serving a specific function.
Resilient Solutions
Orchestration tools manage the lifecycle of components, handling tasks such as resource management, load balancing, scheduling, restarts after internal failures, and provisioning and deploying resources to server cluster nodes. According to the 2023 annual survey conducted by the Cloud Native Computing Foundation, cloud-native technologies, particularly Kubernetes, have achieved widespread adoption within the cloud-native community. Kubernetes continues to mature, signifying its prevalence as a fundamental building block for cloud-native architectures.
Security-First Approach
Cloud-native culture integrates security as a shared responsibility throughout the entire IT lifecycle. Cloud-native promotes security shift left in the process. Security must be a part of application development and infrastructure right from the start and not an afterthought. Even after product deployment, security should be the top priority, with constant security updates, credential rotation, virtual machine rebuilds, and proactive monitoring.
Is Cloud-Native Right for You?
There isn't a one-size-fits-all strategy to determine if becoming cloud-native is a wise option. The right approach depends on strategic goals and the nature of the application. Not every application needs to invest in developing a cloud-native model; instead, teams can take an incremental approach based on specific business requirements.
There are three levels to an incremental approach when moving to a cloud-native environment.
Infrastructure-Ready Applications
It involves migrating or rehosting existing on-premise applications to an Infrastructure-as-a-Service (IaaS) platform with minimal changes. Applications retain their original structure but are deployed on cloud-based virtual machines. It is always the first approach to be suggested and commonly referred to as "lift and shift." However, deploying a solution in the cloud that retains monolithic behavior or not utilizing the entire capabilities of the cloud generally has limited merits.
Cloud-Enhanced Applications
This level allows organizations to leverage modern cloud technologies such as containers and cloud-managed services without significant changes to the application code. Streamlining development operations with DevOps processes results in faster and more efficient application deployment.
Utilizing container technology addresses issues related to application dependencies during multi-stage deployments. Applications can be deployed on IaaS or PaaS while leveraging additional cloud-managed services related to databases, caching, monitoring, and continuous integration and deployment pipelines.
Cloud-Native Applications
This advanced migration strategy is driven by the need to modernize mission-critical applications. Platform-as-a-Service (PaaS) solutions or serverless components are used to transition applications to a microservices or event-based architecture.
Tailoring applications specifically for the cloud may involve writing new code or adapting applications to cloud-native behavior. Companies such as Netflix, Spotify, Uber, and Airbnb are the leaders of the digital era. They have presented a model of disruptive competitive advantage by adopting cloud-native architecture. This approach fosters long-term agility and scalability.
Ready to Dive Deeper?
The Cloud Native Computing Foundation (CNCF) has a vibrant community, driving the adoption of cloud-native technologies. Explore their website and resources to learn more about tools and best practices.
All major cloud providers have published the Cloud Adoption Framework (CAF) that provides guidance and best practices to adopt the cloud and achieve business outcomes.
Final Words
Cloud-native architecture is not just a trendy buzzword; it's a fundamental shift in how we approach software development in the cloud era. Each migration approach I discussed above has unique benefits, and the choice depends on specific requirements. Organizations can choose a single approach or combine components from multiple strategies. Hybrid approaches, incorporating on-premise and cloud components, are common, allowing for flexibility based on diverse application requirements.
By adhering to cloud-native design principles, application architecture becomes resilient, adaptable to rapid changes, easy to maintain, and optimized for diverse application requirements.
We Provide consulting, implementation, and management services on DevOps, DevSecOps, DataOps, Cloud, Automated Ops, Microservices, Infrastructure, and Security
Services offered by us: https://www.zippyops.com/services
Our Products: https://www.zippyops.com/products
Our Solutions: https://www.zippyops.com/solutions
For Demo, videos check out YouTube Playlist: https://www.youtube.com/watch?v=4FYvPooN_Tg&list=PLCJ3JpanNyCfXlHahZhYgJH9-rV6ouPro
If this seems interesting, please email us at [email protected] for a call.
Recent Comments
No comments
Leave a Comment
We will be happy to hear what you think about this post