Data Governance Challenges in the Age of Generative AI
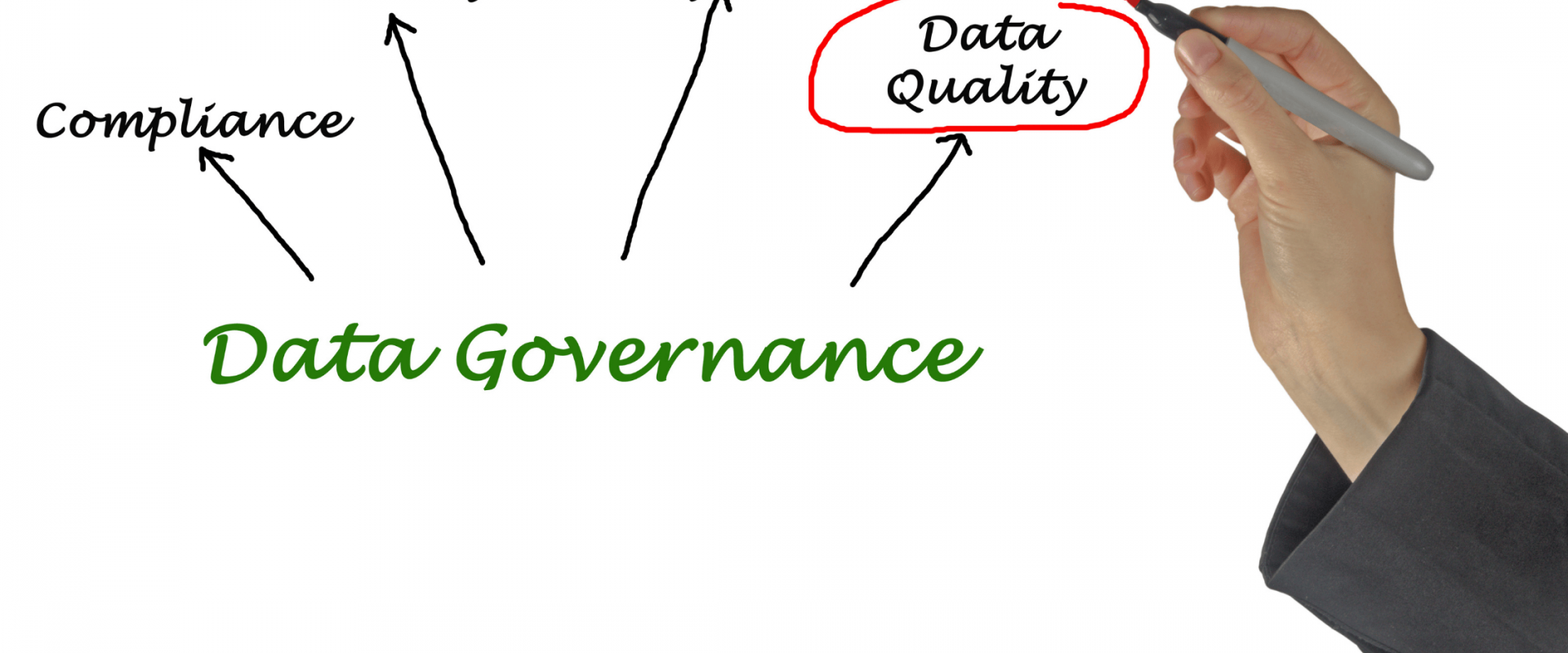
In today’s rapidly evolving technological landscape, Generative AI is transforming industries like finance, healthcare, and e-commerce. However, with great innovation comes great responsibility. Organizations must navigate complex privacy, security, and compliance challenges to ensure effective data governance. This blog explores the intersection of data governance and Generative AI, highlighting key challenges and strategies to address them.
What is Data Governance?
Data governance refers to the policies and processes that ensure the management, integrity, and security of organizational data. Traditional frameworks like DAMA-DMBOK and COBIT focus on structured data management and standardizing processes (Otto, 2011). While these frameworks are foundational, they often lack the flexibility needed for AI applications that process unstructured data types (Khatri & Brown, 2010).
Generative AI: An Overview
Generative AI technologies, including models like GPT, DALL·E, and others, are revolutionizing industries by generating text, images, and code based on large datasets (IBM, 2022). However, these advancements pose unique governance challenges, particularly when handling vast, diverse, and unstructured datasets.
The Intersection of Data Governance and Generative AI
Generative AI impacts data governance by altering how data is collected, processed, and utilized (Gartner, 2023). Managing unstructured data—such as media files and PDFs—is crucial, as it doesn’t fit traditional governance models due to its schema-less nature. Without effective management, AI applications risk mishandling sensitive data, leading to security breaches and compliance failures.
Key Challenges in Data Governance with Generative AI
1. Data Privacy and Security Risks
Generative AI systems process vast amounts of data, often including sensitive information. Without robust security measures, organizations face significant risks of data exposure and breaches. Legal frameworks like the General Data Protection Regulation (GDPR) and the California Consumer Privacy Act (CCPA) mandate stringent data privacy standards, necessitating advanced governance strategies (European Union, 2018; CCPA, 2020).
2. Ethical and Compliance Issues
The use of Generative AI raises ethical concerns, such as biases in AI outputs and data manipulation. Compliance challenges arise as organizations attempt to align AI operations with existing regulatory frameworks, which were not designed for AI complexities (IBM, 2022). New governance models must integrate ethical standards and compliance checks into AI development processes.
3. Quality Control and Data Integrity
Quality control is crucial to ensure AI-generated outputs are reliable. Tools like AWS Glue, Google Cloud’s Data Quality features, and Microsoft Azure Data Factory are essential for maintaining data integrity. These platforms offer capabilities like data profiling and quality scoring, helping organizations monitor and enhance data quality.
Strategies for Effective Data Governance in the Age of Generative AI
1. Policy and Framework Development
Organizations must develop AI-specific policies that integrate data privacy, security, and compliance considerations. For example, masking Personally Identifiable Information (PII) using hashing or redaction techniques, or following field-level encryption, can enhance privacy. Adapting traditional frameworks like DAMA-DMBOK with AI-focused tools can address these challenges.
Modernized tools from cloud providers like AWS Glue and Amazon Macie help with data privacy. Most AWS services are designed to comply with the geographical region where they are deployed, ensuring adherence to data residency requirements.
2. Technological Solutions
Using AI and ML technologies to automate governance processes is vital. Platforms like AWS, Google Cloud, and Microsoft Azure offer advanced tools for managing AI data and ensuring compliance (Gartner, 2023). Implementing these solutions enhances the efficiency and security of data governance practices.
Data quality and enrichment solutions are also critical. Malformed data ingested into Generative AI frameworks can cause large language models to hallucinate. Tools like AWS Glue or Informatica provide data quality scores, offering better context to Generative AI models. Data enrichment solutions, such as synthetic data generation and entity resolution, help avoid bias and toxicity in AI outputs.
3. Continuous Monitoring and Auditing
AI-based monitoring tools enable real-time tracking of data usage and potential security threats, allowing organizations to respond swiftly to anomalies. Regular audits using automated tools like AWS Audit Manager or Azure Purview ensure compliance with governance policies, promote transparency, and highlight areas for improvement.
4. Data Integration and Interoperability Solutions
Investing in a unified data management platform that consolidates various data sources—such as data lakes and warehouses—ensures consistency and compliance across AI systems. Tools like AWS Glue Data Catalog, Azure Data Catalog, and Google Cloud Data Catalog provide functionality for cataloging unstructured data, enabling better discoverability and integration.
5. Cross-Functional Teams and Collaboration
Building cross-functional teams that include data scientists, IT specialists, compliance officers, and business leaders is crucial for aligning data governance strategies with business goals and regulatory requirements. Engaging external stakeholders, like regulators and industry experts, helps organizations stay informed about newer regulations and best practices.
Conclusion
The successful implementation of data governance initiatives for Generative AI establishes a robust foundation for secure data management and machine learning. By integrating AI-specific policies, advanced management tools, and continuous monitoring, organizations can safeguard data assets while ensuring flexibility in production environments.
At ZippyOPS, we provide consulting, implementation, and management services on DevOps, DevSecOps, DataOps, Cloud, Automated Ops, AI Ops, ML Ops, Microservices, Infrastructure, and Security Services. Our proven expertise ensures that your organization is equipped to tackle the challenges of Generative AI and data governance.
Explore our services: ZippyOPS Services
Discover our products: ZippyOPS Products
Learn about our solutions: ZippyOPS Solutions
Watch our demo videos: YouTube Playlist
If this seems interesting, please email us at [email protected] for a call.
By leveraging the right tools, frameworks, and strategies, organizations can navigate the complexities of Generative AI while ensuring robust data governance. Let ZippyOPS be your partner in this transformative journey
Recent Comments
No comments
Leave a Comment
We will be happy to hear what you think about this post